Artificial intelligence (AI) in personalization is changing the landscape of user experiences (UX).
We live in an abundance of information today. As a way to help people navigate this overload, AI services are used as a gamechanger. Much of the information we see online is tailored to our preferences and interactions. Without AI algorithms, we would have found ourselves in the middle of nowhere.
In this article, we will look over the various key personalization techniques that make use of AI systems. In addition to this we will look at how we can apply them in different industries, as well as their distinctive benefits and challenges.
What is AI personalization?
AI personalization refers to the use of algorithms and data to tailor experiences, products, or services to users. To achieve this, the AI system requires data on user preferences, behavior, and interactions. This approach enables the platform to provide relevant and customized content for its targeted users, which leads to higher rates of engagement and satisfaction.
What are AI personalization techniques?
AI personalization techniques are also called recommender systems, or recommendation systems. Different techniques are available depending on the purpose of the personalization. Here are a few:
Content-based filtering
Content-based filtering relies on the characteristics of items to recommend similar items to users. For example, if a user likes a particular movie, the system will recommend other movies with similar attributes such as genre, theme, or actors. This technique uses item features and user profiles to generate recommendations.
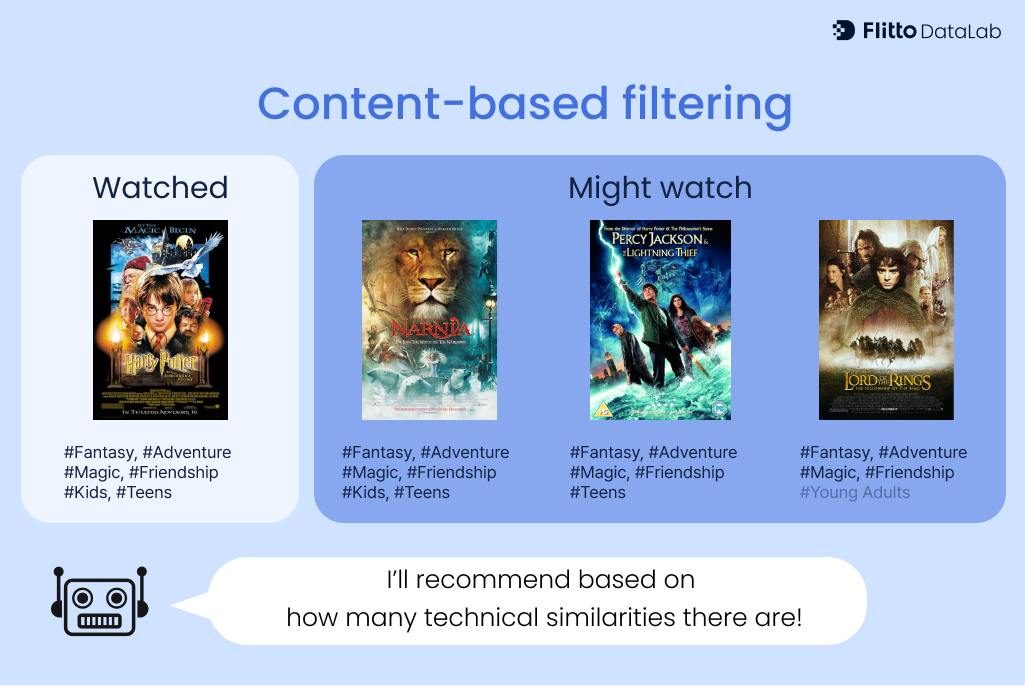
Collaborative filtering
Collaborative filtering predicts a user’s interests by analyzing preferences and behaviors of similar users. There are two types of collaborative filtering: User-based and item-based.
User-based collaborative filtering refers to similar users to determine the items to recommend. It identifies users with similar tastes and suggests items that those users have liked, assuming that the current user will also enjoy those items. This approach can effectively help another similar user discover items that they might not find on their own.
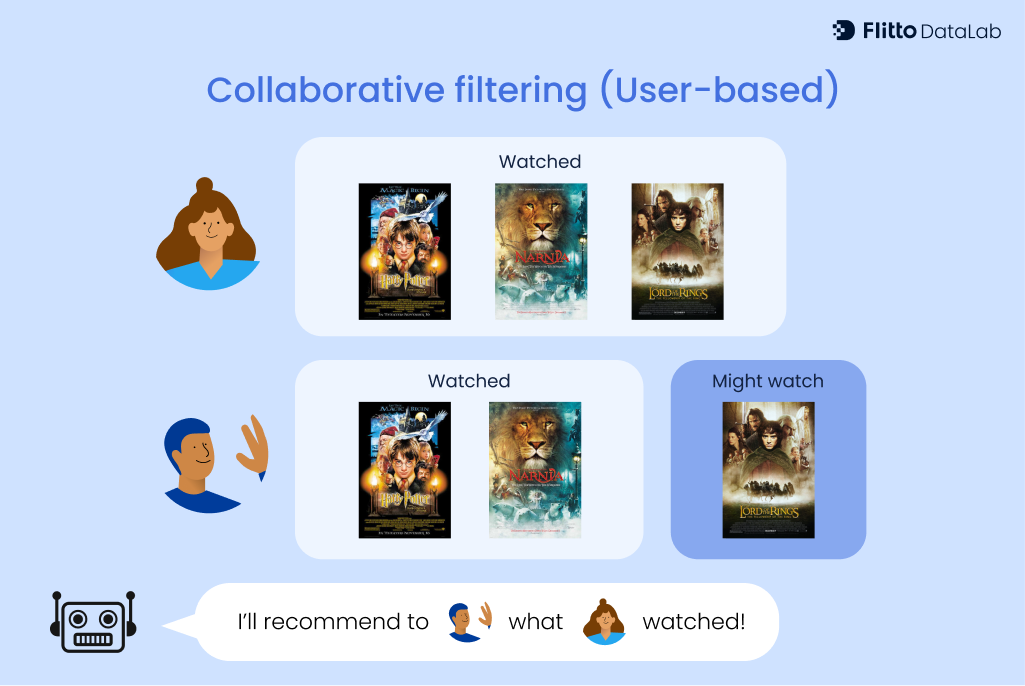
Meanwhile, item-based collaborative filtering recommends items based on the similarity between items themselves. It looks at the patterns of users who have interacted with similar items and suggests items that are frequently liked or chosen together, assuming the current user will like them too. This method is effective in finding related items that naturally cluster together.
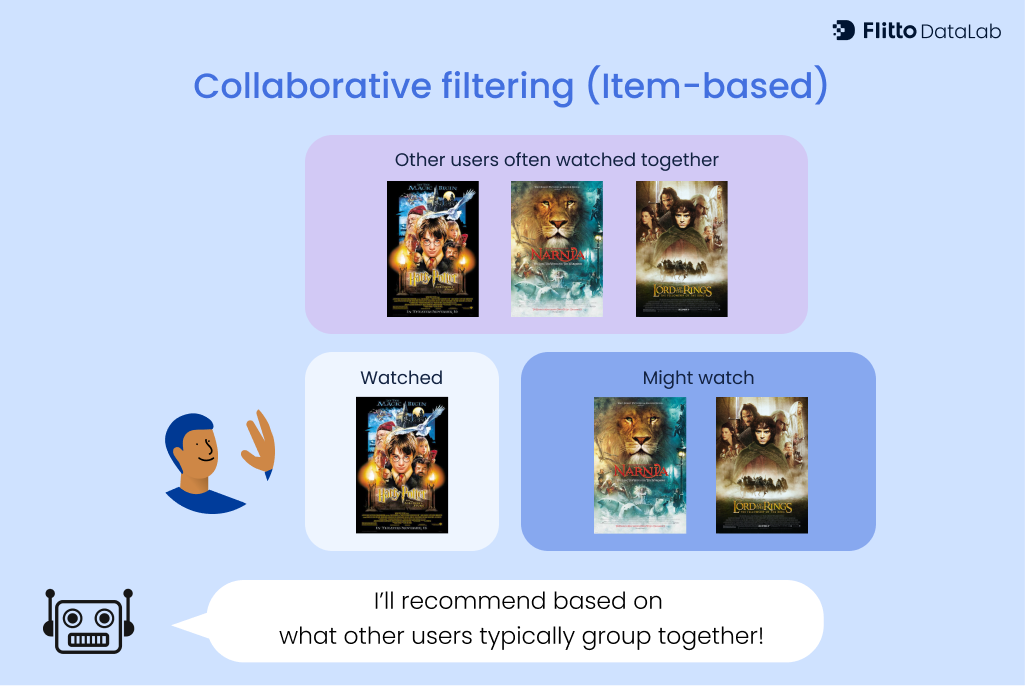
Natural language processing (NLP)
NLP enables AI systems to understand and interpret human language, allowing for more personalized interactions. Techniques like sentiment analysis, entity recognition, and topic modeling help in understanding user preferences and delivering relevant content.
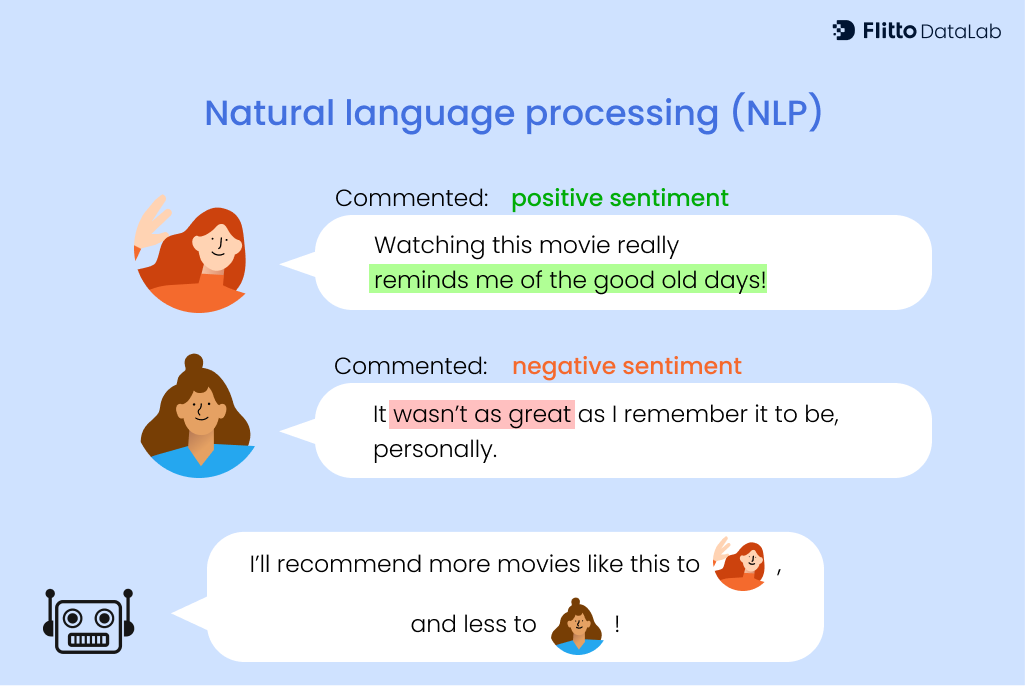
Applications of AI personalization
AI personalization techniques are applicable in different industries, not limited to the examples below. To name a few instances, here are some industries where personalization can matter significantly:
E-commerce
In e-commerce, personalization enhances customer experience by recommending products based on browsing history, purchase behavior, and preferences. In particular, techniques like collaborative filtering and NLP are used to suggest products, offer personalized discounts, and create tailored marketing campaigns.
Healthcare
Personalization in healthcare involves using AI to tailor treatment plans, recommend preventive measures, and provide personalized health insights. AI algorithms analyze patient data, medical history, and lifestyle factors to deliver customized healthcare solutions.
Entertainment, content and media
Streaming services like Netflix and Spotify use AI personalization to recommend movies, TV shows, and music based on user preferences and history. Specifically, these platforms use hybrid filtering techniques to enhance user engagement and satisfaction.
What are the benefits and risks of AI personalization?
There are various benefits in providing personalized experiences through AI systems.
Benefits
When used in the right place, AI personalization can:
- Enhance user experience. Personalized content and recommendations improve user satisfaction and engagement.
- Increase conversion rates. Targeted marketing campaigns and product recommendations can help users move forward with their purchase or consumption.
- Improve customer loyalty. Personalized experiences can help customers feel appreciated and understood, fostering stronger relationships between brands and customers.
- Make decision making easier. AI-driven personalization helps users make informed decisions quickly by presenting relevant options.
Risks
While AI personalization offers numerous benefits, it also presents challenges and ethical concerns.
- Data privacy and security: Collecting and analyzing user data raises privacy issues. Ensuring data security and compliance with regulations like GDPR is crucial.
- Bias and fairness problems: AI algorithms can perpetuate biases present in training data, leading to unfair or discriminatory outcomes. For instance, AIs can assume a certain gender or racial profile prefers a certain product over the other and inappropriately recommend it. To avoid these circumstances, ensuring fairness and transparency in AI systems is essential.
- Over-personalization: Excessive personalization can make users feel uncomfortable or manipulated. To avoid this, it is crucial to balance the personalization so that the user can still feel autonomous about their decisions.
Conclusion
AI personalization techniques have transformed the way businesses interact with their customers, offering tailored experiences that enhance satisfaction and engagement. Moreover, by leveraging content-based filtering, collaborative filtering, NLP, and contextual personalization, companies can deliver relevant and customized content across various industries.
However, for AI personalization to be relevant and helpful for everyone, it is essential to address the challenges and ethical considerations associated with it.
That is where Flitto DataLab can help. Artificial intelligence, like the recommender systems, make use of attribution labels or tags. In doing this, the real-life information must be processed so that AI systems can understand them. Flitto DataLab offers a comprehensive range of datasets to process natural language, and help other AI systems understand human concepts and logics better.
High-quality datasets can enhance AI models’ accuracy and efficiency, ultimately delivering more personalized and effective solutions to their customers. With Flitto DataLab, you can ensure your AI systems are equipped with the data they need to excel in today’s competitive market.