Arabic is a highly challenging language to learn for non-native speakers. It takes a long time just to learn how to read it.
This is where optical character recognition (OCR) technology comes in handy. This technology enables a conversion of printed or handwritten text into machine-readable text. As a result, it can save time and energy, and makes it easier for non-Arabic speakers to understand Arabic texts when hand in hand with other AI technologies like machine translation.
However, Arabic text recognition is also a challenging task for machines, just like it is for humans. There are numerous features in the Arabic language that makes it difficult for machines to detect and recognize texts.
In this article, we’ll look at what makes Arabic text recognition a formidable task for machines and explore some solutions to address it.
What is Arabic optical character recognition (AOCR)?
To understand AOCR engines, we’ll need to understand what the general OCR engines do. OCR tasks typically encompass two core problems: text detection, which identifies text samples within given images, and text recognition, where algorithms decipher and transcribe the identified text into a language that humans can understand.
Arabic optical character recognition (AOCR) is an interesting subfield of OCR technology that specializes in Arabic texts. Like other OCR engines, AOCR can learn to recognize handwritten and printed texts with appropriate datasets.
Interestingly, there is a separate dedicated research on building an OCR for Qur’an, the holy book of Islam. This is due to the highly artistic and rich Arabic calligraphy used in the sacred book.
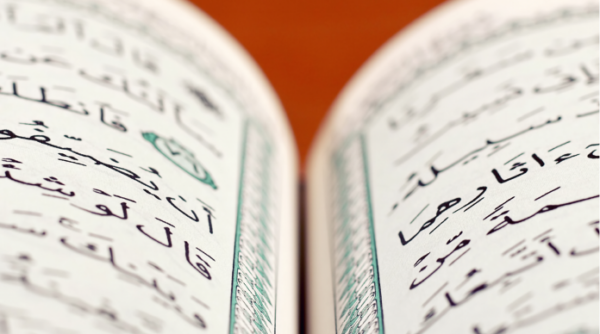
When do we use Arabic text recognition systems?
The applications of OCR are diverse, spanning document scanning, automated indexing, and form processing. It’s helpful in any field where you need to edit, search, or index texts.
The range of applications for Arabic text recognition is as broad as any other languages. It includes:
- Banking: OCR is widely employed in banking services. For instance, it can swiftly process check payments by scanning and transferring checks within seconds.
- Healthcare: In healthcare, OCR makes it easy to transfer and index diverse patient data into databases.
- Communication: Arabic text recognition system, together with machine translation systems, enables non-speakers of the language to understand Arabic texts.
Moreover, AOCR can assist in preserving a significant amount of cultural heritage, particularly the literary works. This process involves having to convert physical copies of historical literary texts and scanned page images into searchable full text for future access. Further advancements in Arabic text recognition will enable researchers to substantially decrease the expenses associated with this process, both in terms of time and financial resources.
What makes Arabic OCR engines difficult to train?
Arabic, also known as the language of God, is the fourth most commonly spoken language in the world. It is spoken by more than 433 million people globally and serves as an official language in 26 countries.
Meanwhile, there are multiple reasons that make training Arabic OCR engines challenging.
First, the Arabic language features inherently challenging traits. These traits introduce various hurdles in training the system, especially in terms of segmentation, character overlap, and contextual dependencies. These are all crucial aspects in the OCR mechanism.
Here are some notable features in the Arabic language:
- Arabic is a right-to-left (RTL) language (many of the currently available models are based on the left-to-right (LTR) writing system).
- There are at least 81 shapes used in the Arabic writing system. Some of these letters share similar shapes, distinguishable only by dots.
- Arabic is written in cursive, which means there are no default spaces between characters.
- Some characters change their forms in relation to its position.
- Handwritten texts could vary greatly per person.
Arabic handwritten text is especially noted to have a broad range of “acceptable” versions. Native speakers tend to omit diacritics when writing, and use various shortcuts to make writing easier.
Let’s look at some examples of Arabic handwritten texts. The following images contain words that can be written in various ways, depending on the writer’s preferences.
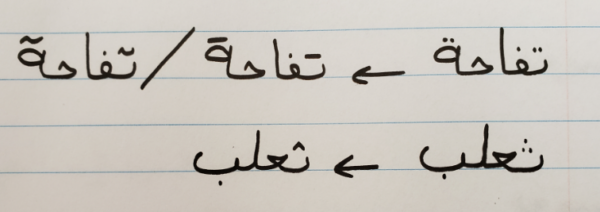
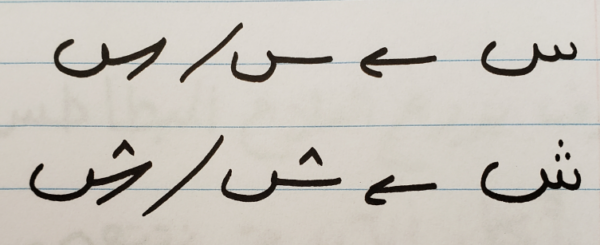
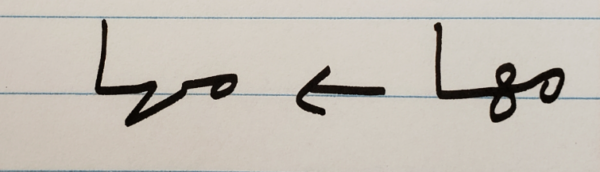
The two text images below contain identical sentences. Notice the explicit differences between them:
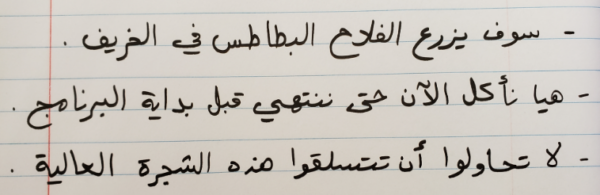
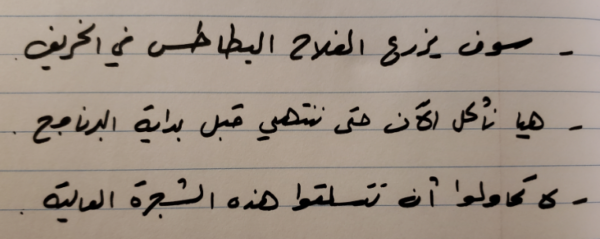
What are the current challenges in Arabic text recognition?
There are multiple stages in training an OCR engine, like image collection, pre-processing of data, segmentation, feature extraction, and classification.
However, one of the main issues in Arabic text recognition is that there is a notable absence of solutions that encompass the entire process, from segmentation to post-processing. Much of the currently research primarily concentrates on the recognition phase alone.
Moreover, most attempts in developing Arabic OCR have focused on the general applications purposes. Arabic handwritten recognition has received relatively limited attention.
The most important issue is that there is a shortage of comprehensive and diverse datasets for Arabic handwritten recognition. This imposes limitations on the development of large deep learning models for addressing the challenge of OCR with Arabic handwritten fonts.
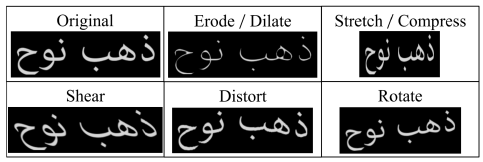
Synthetic datasets are useful during the earlier stages of the process. In addition to that, we need different types of datasets in order to address the current challenges.
Types of Arabic text recognition datasets
Arabic text recognition engines, or AOCR, share similar subsets of datasets necessary to train the typical OCR engines. Which type to choose depends on the use case.
There are two broad subsets of Arabic OCR data: Arabic handwritten text data and Arabic printed text data. Each subset comprises another two variants: online and offline.
Here are some examples in each subset:
- Offline handwritten text data: Images of handwritten letters, manually filled-up documents, students’ test papers, calligraphy
- Online handwritten text data: Handwritten notes on tablet PCs
- Offline printed text data: Printed documents, restaurant menu, street signs, nutritional facts on food products
- Online printed text data: Images with rasterized texts
An interesting fact: researchers have found that you can also use the datasets of other languages that are linguistically similar, like Urdu, to improve performance in Arabic OCR systems.
Urdu and Arabic exhibit numerous similarities. They share identical writing styles characterized by a cursive nature and the RTL writing system. While Urdu boasts a larger character set with approximately 39 to 40 letters, Arabic has a slightly smaller inventory. Urdu enriches its vocabulary by borrowing extensively from Arabic, accounting for nearly 30% of its words. Due to these linguistic affinities, most Urdu speakers can effectively read the Quran. Consequently, datasets and trained models for both languages are frequently employed.
Several datasets designed for handwritten text recognition in Urdu are accessible. Given the shared vocabulary and alphabet between Urdu and Arabic, leveraging Urdu datasets can yield promising results in Arabic handwritten text recognition.
Conclusion
In this article, we’ve looked at some challenges that exist in training Arabic OCR engines.
Arabic is one of the most difficult languages to learn even for humans. It is not surprising that engines have a hard time too.
We can’t solely rely on web content for language data either. A significant discrepancy exists between the number of Arabic speakers and the amount of Arabic text available online. In fact, this difference is one of the largest among the top 20 most used languages in the world.
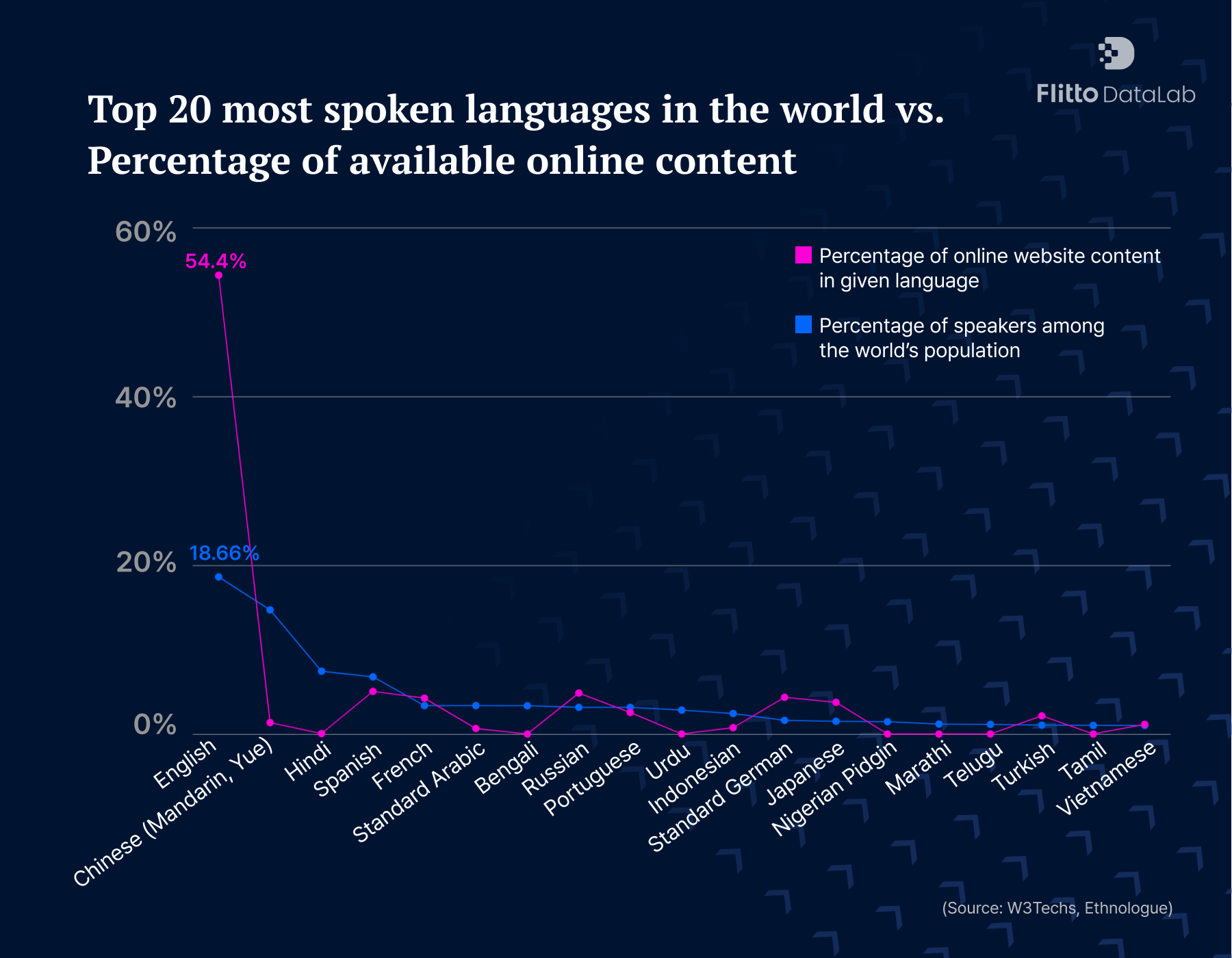
This means that if you were to train a text engine solely by scraping online content, you would soon encounter an inherent limitation.
The diversity and scale of datasets will be a crucial factor when it comes to overcoming the current challenges in OCR benchmarks.
Flitto DataLab offers scalable machine learning data solutions across diverse languages, powered by a 13M user platform.
Visit our page on OCR data solutions, and feel free to check out some of our sample OTS datasets.